3.3 Passenger Vehicles
The passenger vehicles model in REGEN characterizes the fleet composition and energy use associated with light-duty vehicles for personal transportation. Like the buildings model, the passenger transport model begins by using detailed data to allocate service demand by technology within modeled household structural classes. Data for passenger vehicles come primarily from the 2009 National Household Travel Survey (NHTS), which is a U.S. Department of Transportation Federal Highway Administration dataset that provides comprehensive historical information on travel and transportation patterns in the United States.
Regional data breaking down households into structural classes, as well as the average driving intensity and age distribution of vehicles, are based on NHTS data. The model distinguishes between households of different settlement types (with three classes for urban, suburban, and rural locations), driving intensities (with two classes based on annual miles driven), and the number of cars owned.
The model projects total service demand by time and model region based on household growth rates (which are constructed from the same population projections as the building model). Exogenous assumptions about the turnover of the existing fleet are based on vintage estimates from the NHTS dataset and assumed survival rates of vehicles. Scrappage lifetimes for vehicles come from the Bandivadekar, et al. (2008), "On the Road in 2035" study. The model tracks turnover and purchase decisions for each household, and each decision accounts for the number of vehicles in the household alongside the composition of primary and secondary vehicles.
Next, the passenger vehicles model assesses the total cost for discrete technological options in each vintage across the explicit structural classes. Vehicle types include internal combustion engine vehicles (ICEVs), battery electric vehicles (EVs), plug-in hybrid electric vehicles (PHEVs), and a vehicle subscription (i.e., mobility-as-a-service) option. The model includes a bottom-up description of the costs and operations of alternate vehicle choices and characterizes the trade-offs from the consumer perspective. The logit nesting structure is shown in Figure 3‑13. Again, costs within each category vary by vintage, region, and other structural classes across the technologies. To assess the total costs of each option, the model calculates the sum of the vehicle purchase price (including home charging equipment) and operating costs (including retail fuel costs, operations, maintenance, insurance). Costs of home charging equipment are based on EPRI estimates and vary by vehicle and settlement type (ranging from $0 to $1,500).
Since vehicle purchases are complex, multi-attribute decisions, household decisions also include behavioral costs associated with consumer preferences about range anxiety, refueling, and vehicle availability, which can change over time. Availability represents the implicit cost of consumers not being able to find an EV or PHEV in their preferred size class, and these costs decrease to zero by 2030. Refueling costs for ICEVs, EVs, and PHEVs (from $0 to $1,800) and range anxiety costs for EVs (from $0 to $5,800) vary by settlement type, driving intensity, and configuration of other household vehicles and are based on the Market Acceptance of Advanced Automotive Technologies (MA3T) model from the Oak Ridge National Laboratory.
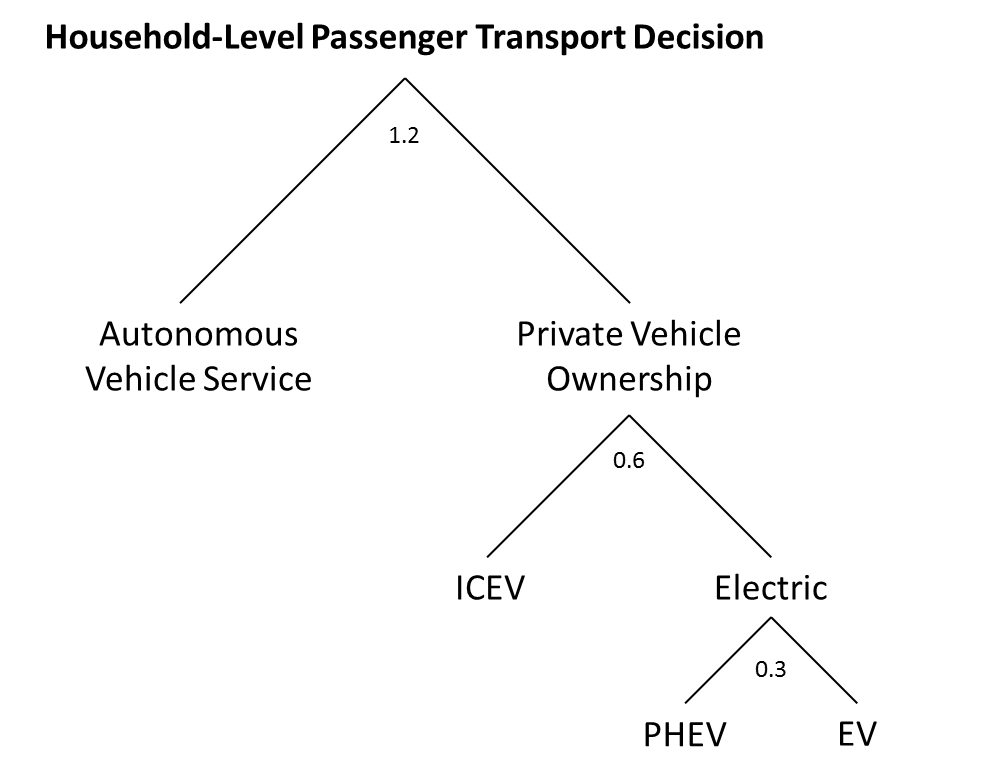
New vehicle cost and performance assumptions are shown in Figure 3‑14. Costs come from EPRI and Argonne National Laboratory estimates. Figure 3‑15 compares historical and projected battery cost assumptions, which shows the assumed US-REGEN values relative to other recent publications. The reference assumptions for the PHEV and EV range are 40 miles and 250 miles, respectively. Fleet average fuel economy is also shown in Figure 3‑14. EV and PHEV efficiencies assume that the relative size of the vehicle class is increasing over time, which reflects consumer preferences for larger model types. The model accounts for aggregate trends in vehicle sizes but does not include structural modeling of customer segments across vehicle size classes. Charging efficiencies for electric vehicles are assumed to increase from 85% in 2015 to 94% in 2050. Efficiency improvements for ICEVs reflect assumed levels of hybridization, which increases from 50% in 2030 to 95% in 2050.
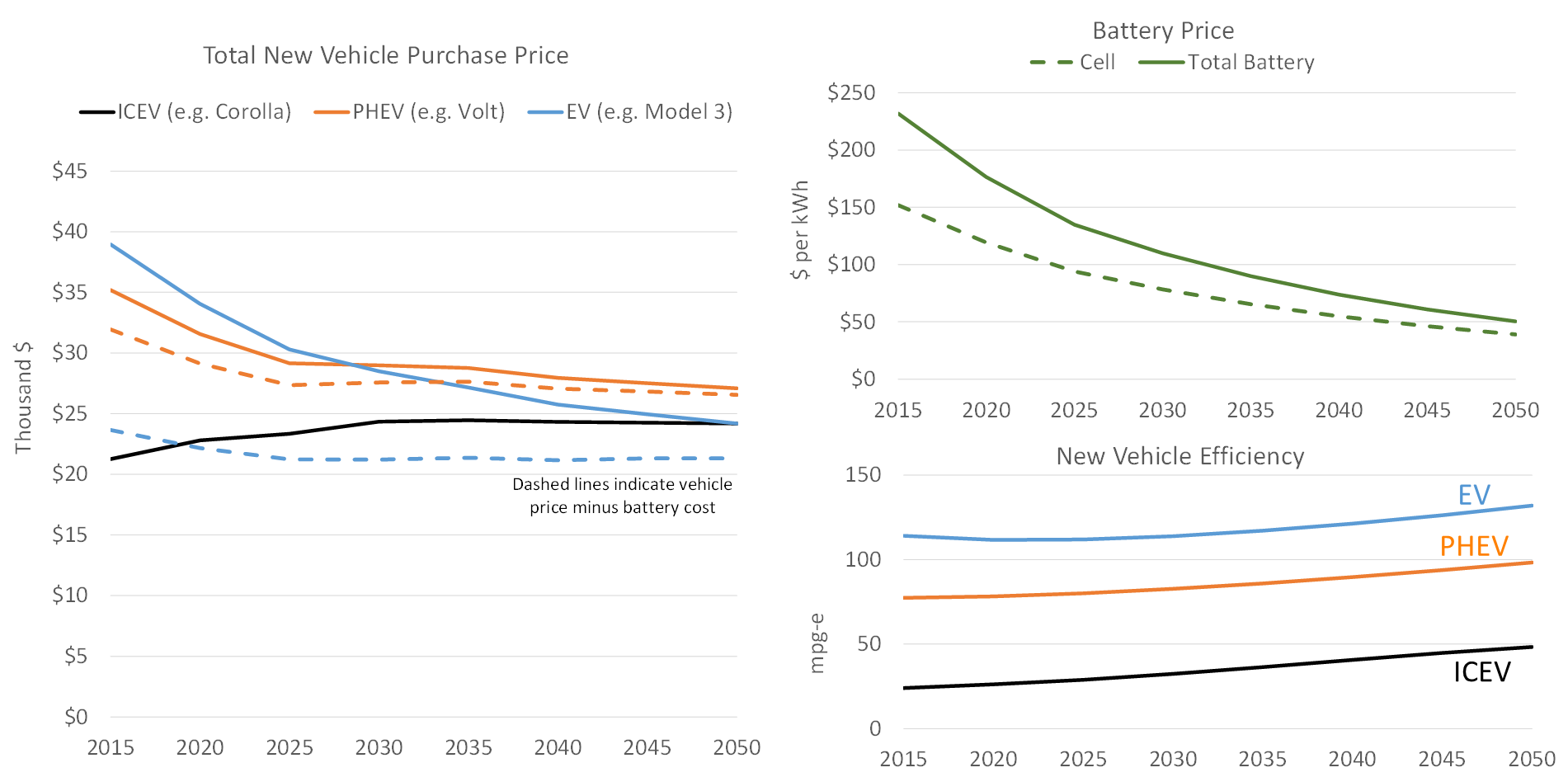
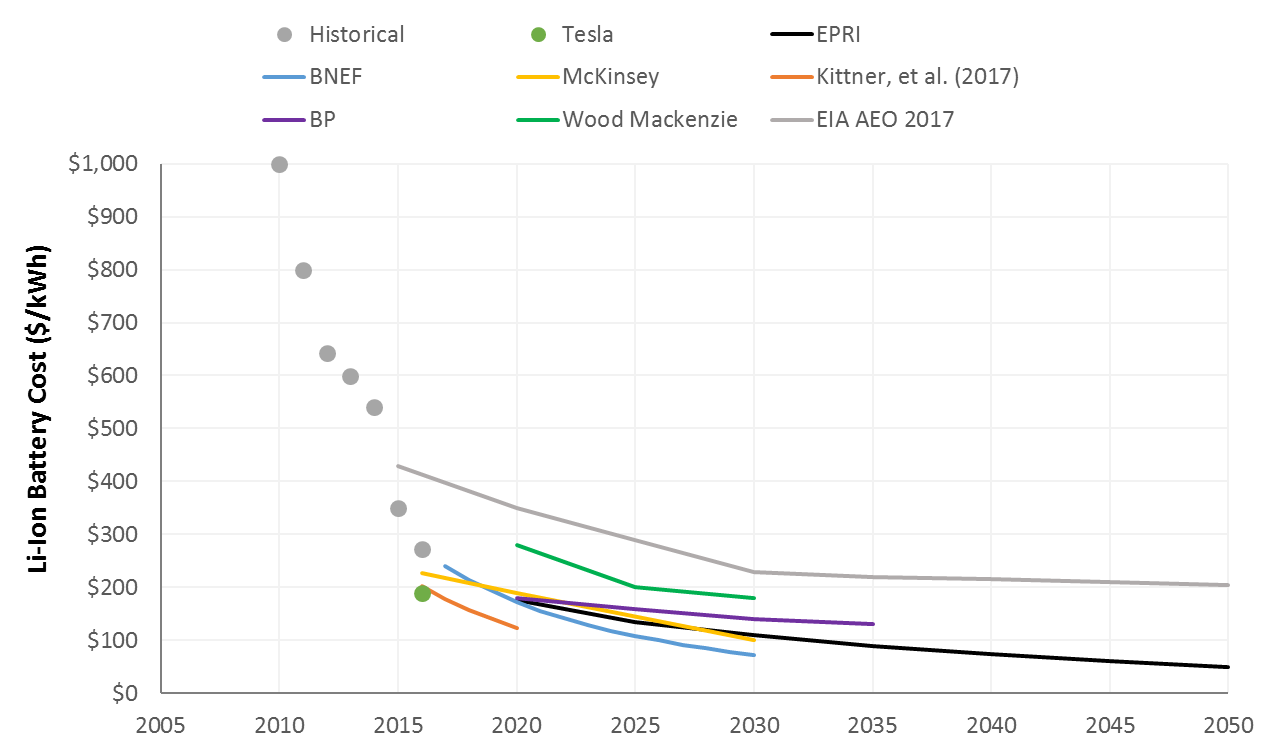
3.3.1 Mobility-as-a-Service and Autonomous Vehicles
While technical, safety, and regulatory hurdles remain for deployment at scale, the potential economic advantages of mobility-as-a-service options and autonomous vehicles—essentially replacing labor with relatively less expensive capital in the form of sensors and software—are spurring significant investment and strategic shifts throughout the industry. As shown in Figure 3‑13, REGEN can represent an autonomous-based mobility service that competes directly with the traditional mode of private vehicle ownership for passenger transport. The economic potential for ridesharing and driverless vehicles derives from higher utilization rates, which offer strong synergies with a low-operating cost electric battery platform. Many low-annual-mileage drivers, particularly in urban environments with high fixed costs of vehicle ownership (such as parking and insurance), would have much lower overall costs using a service than by owning a private vehicle.
In addition to autonomous vehicle services competing against privately owned vehicle purchases, REGEN has an autonomous vehicle service model that includes decision variables for the number of vehicles of alternative technology types, including both EVs and ICEVs, number of EV chargers at various speeds, and dispatch of vehicles (and chargers) against an hourly demand profile for vehicle miles traveled. The model is solved as an optimization of vehicle and charging infrastructure capital costs plus fuel and operating costs of vehicle dispatch. The scale of service demand is normalized, as all costs are linear, so that the resulting solution yields an average price per mile for the autonomous vehicle service based on assumptions about costs, fuel prices, and capacity utilization which is a function of the demand profile. This price is used as the input to a vehicle choice model at the household level, in which one option for vehicle purchase is to instead "subscribe" to the autonomous service at the model's calculated price per mile. These components are solved iteratively with a model electric supply, and in scenarios with real-time pricing for the autonomous vehicle fleet, the hourly price is applied as input to the service model's optimization of charging profile.
To account for additional vehicle miles and energy consumption needed to originate passenger trips (known as "deadheading"), the model includes a proportional increase on top of the exogenous assumption for service demand. However, REGEN does not include other potential rebound effects, such as increased demand for trips or higher occupancy rates.
3.3.2 Charging Shapes
The passenger vehicle model currently assumes fixed charging shapes for different household types. These profiles are based on the EPRI-NRDC (2015) study "Environmental Assessment of a Full Electric Transportation Portfolio, Volume 2" (EPRI ID 3002006876). As shown in Figure 3‑16, there are three charging profiles, and a mix of these profiles by different household types gives rise to an uncoordinated charging equilibrium. REGEN also provides options for flexible charging as well, including for a fleet of autonomous vehicles.
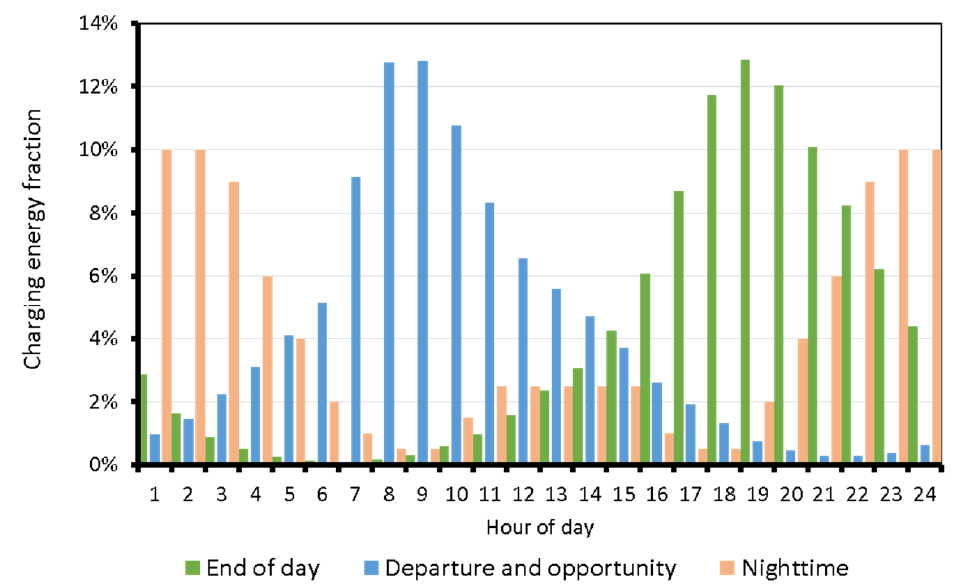