B. Forestry and Agricultural Biomass Supply Modeling
US-REGEN makes use of regional agricultural and forestry biomass supply feedstocks derived from the Forest and Agriculture Sector Optimization Model with Greenhouse Gases (FASOM-GHG).[1] FASOM-GHG is a dynamic economic model of U.S. forestry and agricultural commodity production and markets, including land-use allocation and land management decisions. The model is unique in its detailed simultaneous and dynamic optimization of forestry and agricultural production and markets, and has a long peer-review publication record (e.g., Baker et al., 2010; USEPA, 2005; McCarl and Schneider, 2001). Furthermore, the model has been updated to include simultaneous consideration of possible production and end-use of a broad set of agriculture and forestry residue and dedicated bioenergy feedstocks. The model has also been improved to capture logistics of delivered biomass for energy use (i.e., storage and transportation). The core model development team includes Texas A&M University, Oregon State University, the U.S. Forest Service, RTI International, Duke University, and EPRI. This appendix provides an overview of the model's structure, a discussion of specific bioenergy modeling features, and the US-REGEN implementation.
Model Structure
FASOM-GHG[2] is an economic model that solves for the market equilibrium prices and quantities that simulate inter-temporal market clearing in the U.S. forestry and agricultural sectors given a set of assumptions. It does this by following Samuelson and maximizing the net present value of what economists refer to as the sum of consumer and producer surplus. The model is used to simulate alternative futures and glean insights regarding the potential implications of different assumptions regarding future policy, technology, land productivity, etc.
To model long-run forest investment decisions and long-run policy proposals, model simulations have 5-year time steps and a time horizon of at least 50 years depending on the application. FASOM-GHG typically models eleven major market regions (Figure B-1), but this can vary depending on the application and computational constraints. Model results over time include projections of commodity prices and quantities, production input prices and quantities, export and import quantities, land-use allocation between agriculture and forestry and between specific crops as well as pasture, and land management system choices such as chemical inputs, irrigation, tillage, and crop type.
FASOMGHG models primary and secondary forestry and agricultural commodities, which currently includes approximately 70 primary and secondary crop products, 40 primary and secondary livestock products, 10 categories of forest and agricultural residues, 30 categories of public and private domestic and imported logs, 40 forestry products included in the model as secondary commodities, and 10 processing byproducts. Commodity supply and demand are calibrated to historic price and production data.
Land is allocated within each region to the combination of uses that yields the highest net present value. The productivity of land varies by use and region and is a function of biophysical conditions and growth characteristics, land management, and exogenous technological improvements.
As noted, FASOM-GHG in an inter-temporal optimization model. The same is true for US-REGEN. This means that modeled decisions take into account expectations about future markets. This is frequently misunderstood as implying perfect knowledge about the future. Instead, however, it is a modeling approach that recognizes and attempts to capture the fact that investment decisions today are influenced by expectations about future markets and future returns on investments.
Finally, it is important to note that FASOM-GHG results, like US-REGEN results, are not forecasts or predictions, but instead projections based on a set of assumptions about potential future biophysical, technological, and economic conditions. Both models are market simulation models designed to entertain long-run potential alternative futures and evaluate the potential implications of policy designs in the distant future, where prediction is impractical. The results are meant to inform strategic planning and policy design with insights on potential outcomes and implications. One of the key strengths of simulation models is their ability to assess possible future outcomes under various plausible scenarios, including circumstances that have limited historical data from which to draw (e.g., futures with significant greenhouse gas incentives or bioenergy production goals, and futures with currently non-commercial technologies such as cellulosic ethanol).

Bioenergy Details
The version of FASOM-GHG developed and utilized here includes a variety of conventional dedicated and residue agriculture and forestry biomass feedstocks, as well as bioenergy end-uses (Table B-1). Simultaneous modeling of the set of agriculture and forestry bioenergy feedstocks and end-uses allows us to capture competition and complementarities, as well as the value of co-production. As shown in Table B-1, multiple feedstocks can be used for a bioenergy use and a single feedstock can be used for more than one use.
The relative value of an individual feedstock is a function of direct costs (associated with harvesting, transportation, storage, and processing), the opportunity costs of alternatives, energy content, moisture content, energy prices, co-products (e.g., oil and livestock feed substitutes), and direct and net greenhouse gas benefits if valued. Table B-2 lists the energy (higher heating value) and moisture content assumptions for each feedstock. Note that the feedstocks are assumed to have undergone some natural drying in the field/forest (i.e., not bone-dry, but not just harvested moisture content).
Because of the seasonal nature of the availability of some biomass feedstocks, we include off-site storage to ensure year-round availability. This is in addition to short-term on-site storage capacity at power plants or refineries that is included in the cost of each bioenergy conversion technology. For off-site storage, we have assumed a shrink-wrapped bale technology that is relatively inexpensive with little biomass decomposition (we assume 4% loss to decomposition). The technology has a fixed cost of $6 per ton and monthly cost of $0.35/ton. Table B-3 provides our storage cost assumptions. For example, we assume crop residues can be harvested over two months of the year during the fall. Therefore, a maximum of 10 months of storage is required, and the average storage requirement per ton is 4.6 months. The shrink-wrapped technology costs include unloading and loading costs. Shrink-wrapped bales can be stored on-farm and have modest capital and variable cost requirements, compared to a covered cement pad for example with per ton costs about twice that of shrink-wrapped bales. Off-site storage costs apply regardless of whether the biomass is used for power or transportation.
Like storage, transportation of biomass is a critical component of the cost of biomass fuel per unit energy given the low energy density of biomass compared to fossil fuels. In general, we model the costs of transporting biomass as two stages: farm/forest to collection point, and collection to existing electricity generation units (EGUs). Off-site storage is assumed to occur at the collection points. In addition to proximity (collection to farm/field, and collection to existing generation), which defines distance, transportation costs for each of the feedstocks inTable B-1 are determined by biomass energy yield per ton, spatial density of the biomass being used as a feedstock (defined as the proportion of the land area around a collection point with available biomass of the type being used as a feedstock), and the truck-hauling rate (load size and number of loads required for a given amount of energy). Biomass originating at farms/forests close to existing EGUs is assumed not to go to the collection points. Also, new ethanol refineries are assumed to be located at the collection points, which could be true for new dedicated biomass power plants as well.
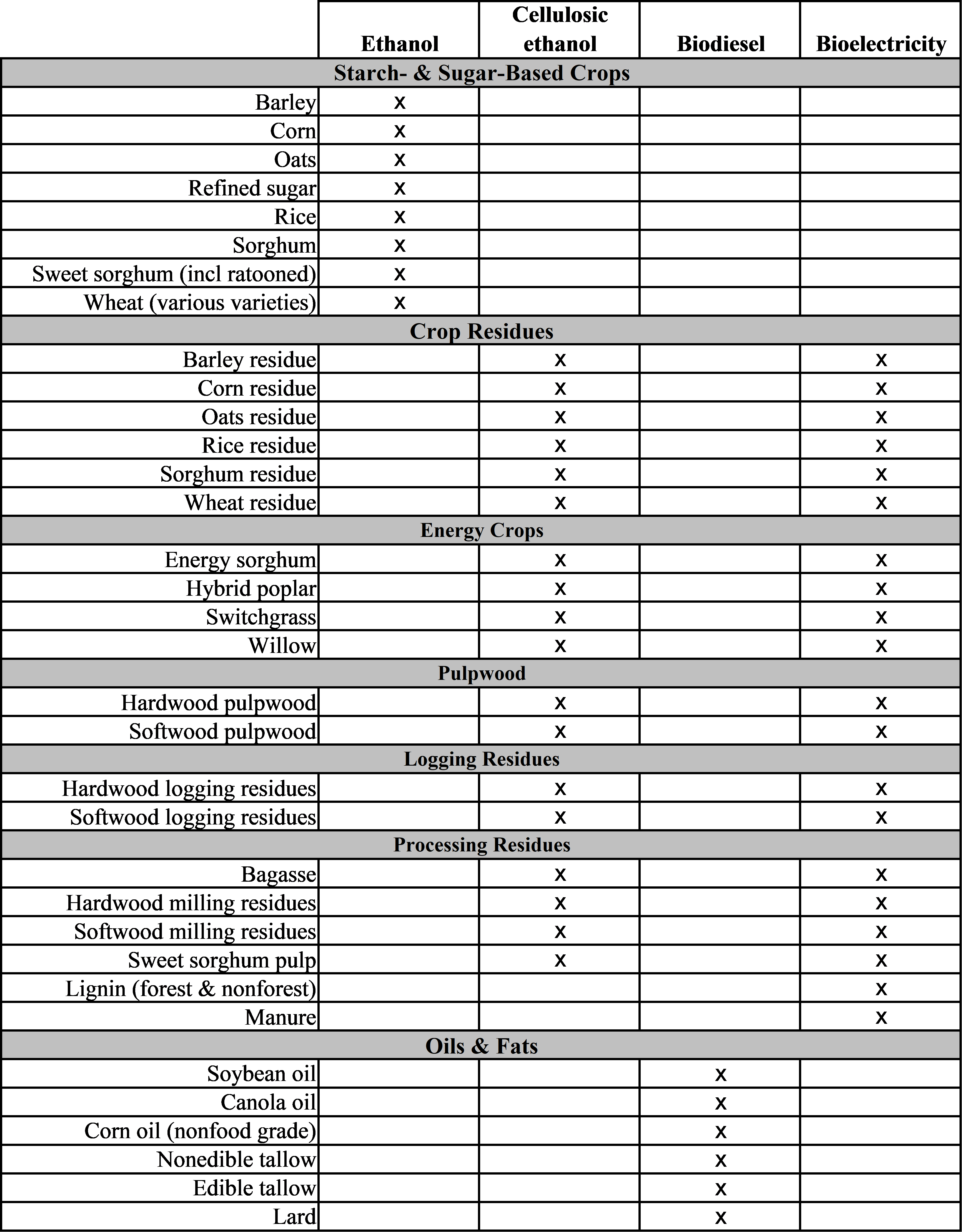
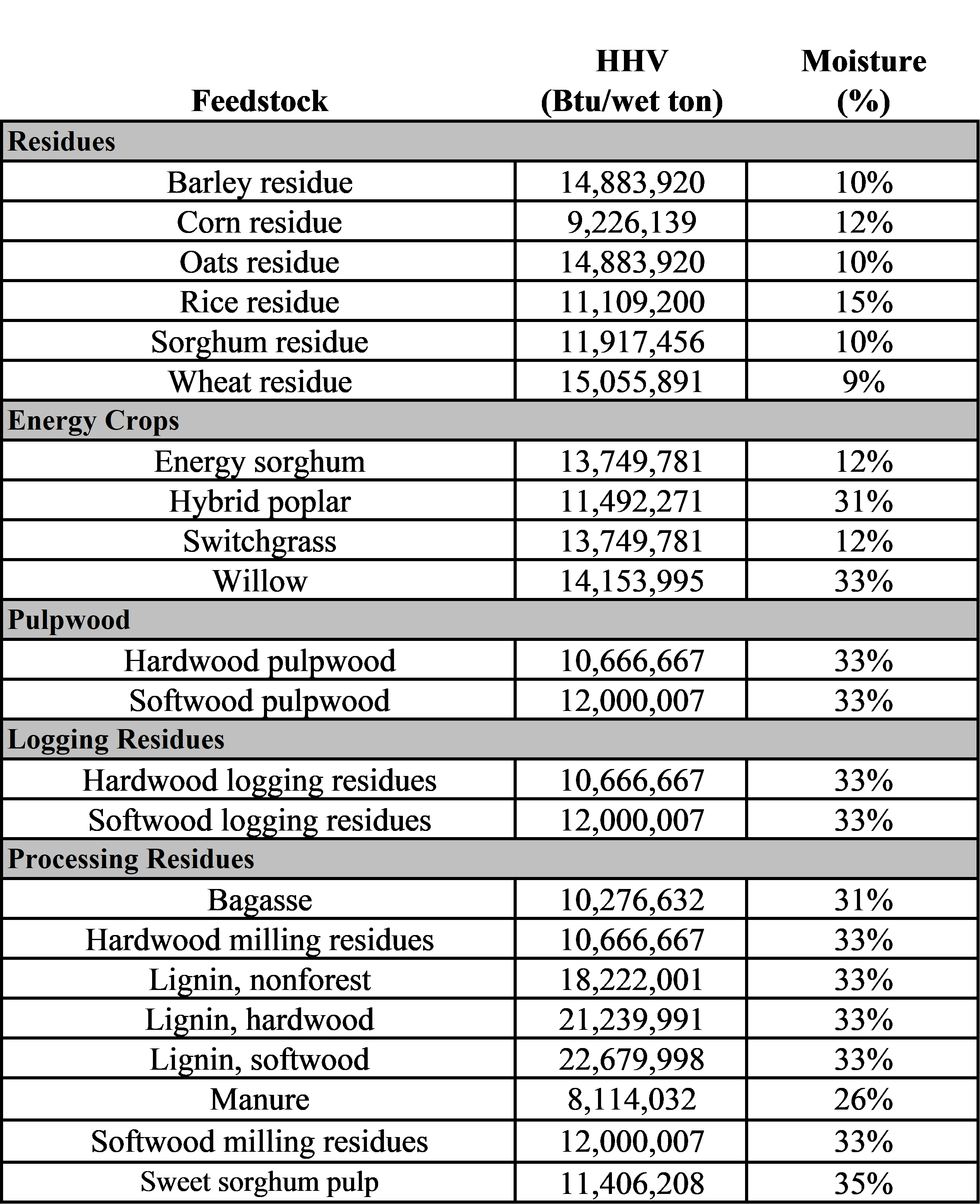
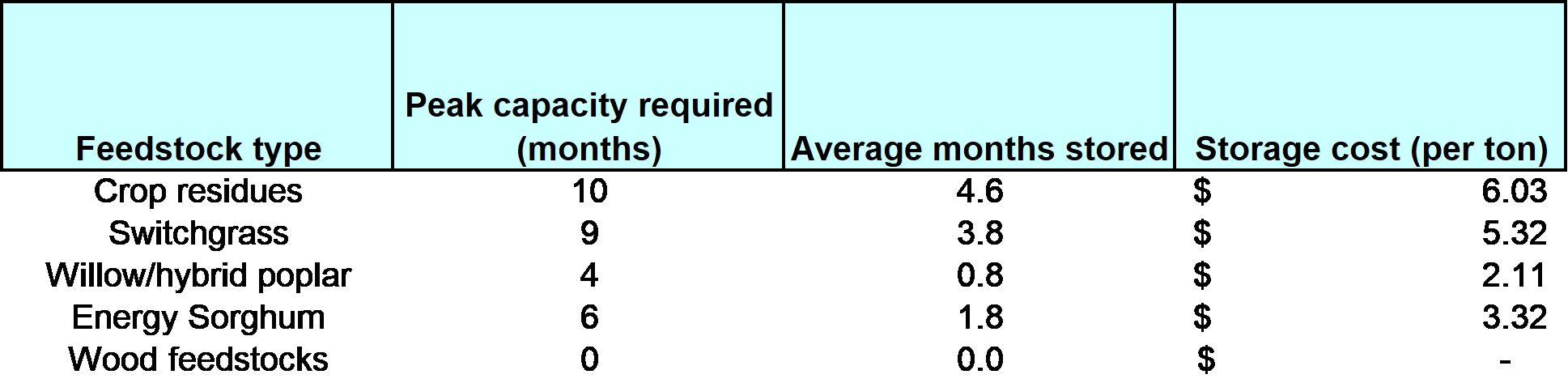
For the first stage, average biomass transportation costs per ton are computed for each region based on an approach developed by French (1960) and described in McCarl et al. (2000) that assumes a gridded road system and considers biomass proximity to county centroids. Fixed cost, cost per mile, and load size for grain crops are assumed to be $45 per load, $1.10 per mile, and 50,000 lbs respectively (converted into the appropriate unit for a given crop to be consistent with the yield measure, for example, bushels). Costs for crop residues and energy crops are assumed to be twice as high at $90 per load and $2.20 per mile. In addition, because of their higher moisture content and lower densities, the average load size for crop residues, sweet sorghum, switchgrass, and energy sorghum is assumed to be 40,000 lbs. For processing residues, there are no hauling costs because they are assumed to be used at the site where they are generated. Similarly, no hauling costs are included in biodiesel production because they are already included in other stages of production where fats and oils are produced.
In the second stage, feedstocks are hauled from collection points to existing power plants with hauling distances calculated based on a GIS assessment of the location of cropped acreage relative to the location of existing generation. Regional hauling distance functions are simultaneously derived for increasing quantities of delivered biomass. In the Corn Belt for instance, which has both large quantities of potential feedstocks and coal-fired plants, the average distance increases slowly—from 14.1 miles for the first 500 MW to 16.7 miles at 15,000 MW and to 32.42 miles at 25,000 MW. In the Pacific Southwest, on the other hand, second-stage hauling distances increase from 15.0 miles for the first 500 MW to 950.9 miles by 1,500 MW because of a combination of lower feedstock availability and less coal-fired generation.
US-REGEN Bioenergy Implementation
We have implemented biomass as a fuel alternative in US-REGEN in two model development phases: first, as a fuel alternative for the electricity sector; and second, as a fuel alternative for industrial and transportation sector use, as well as electricity use. The second phase is currently in progress. In the first phase, industry and transportation demands for biomass were endogenously determined within the FASOM-GHG model, which modeled forest product sector heat and power biomass consumption and biofuel (ethanol and biodiesel) demand for biomass. Biofuel volumes included mandatory compliance with the latest Renewable Fuels Standards (RFS) associated with the U.S. Energy Independence and Security Act. In the second phase, the US-REGEN end-use model will determine the allocation of biomass across sectors internally. Table B-4 illustrates the model structure for the use of bioenergy across sectors and feedstock types. In the macro context, biomass supply can come from agriculture and forestry as well as municipal solid waste and landfill gas. Because historic residential and commercial use of biomass is relatively constant and unlikely to be affected by energy and climate policy, we assume small exogenous and fixed quantities of biomass consumption by those sectors. Conversely, we will model industry, transportation, and electric power bioenergy.
Figure B-2 illustrates our initial approach to macro modeling of bioenergy. With FASOM-GHG, we can develop four types of biomass supply curves: raw biomass for heat and power, and three types of refined biofuel supplies—cellulosic ethanol, conventional ethanol, and biodiesel. Developing biofuel supplies within FASOM-GHG, versus raw biomass that is refined into biofuels in US-REGEN, allows us to take advantage of the biofuel refinery characterizations developed for FASOM-GHG for EPA RFS rulemaking. With this approach, we can have biofuel compete directly at the pump within US-REGEN with refined fossil transportation fuels.
The delivered cost of ethanol includes the increasing systems and lower performance costs associated with E85 penetration. As total ethanol volume increases, increasing levels of E85 will be required due to the E10 blending wall, as well as constraints preventing the penetration of mid-level blending. E85 will increase in level and as a share of total ethanol produced, while E10 total volumes would decrease as total ethanol volume increase. More E85 requires more distribution infrastructure (transportation, distribution, and dispensing) and/or higher Flex Fuel Vehicle (FFVs) refueling rates and stations carrying E85. E85 has a lower return compared to E10 due to E85's lower energy content as well as more limited E85 fueling availability. These issues imply an increasing ethanol penetration cost with increasing total ethanol volume in order to reflect the growing share of E85. We were able to estimate an ethanol penetration cost function from EIA projected differentials in gasoline and wholesale ethanol prices. For instance, with 15 bgal of total ethanol/year we assume an E85 share of 3% and ethanol penetration costs of $0.20/gallon. For 20, 30 and 40 bgal/year, the E85 shares are 16%, 40% and 70% and penetration costs $0.20, $1.00 and $1.40/gallon respectively. Penetration costs max out at $1.80/gallon for volumes greater than 45 bgal/year. Note that penetration costs are in addition to the cost of producing and transporting biomass feedstocks to refineries and conversion to ethanol.
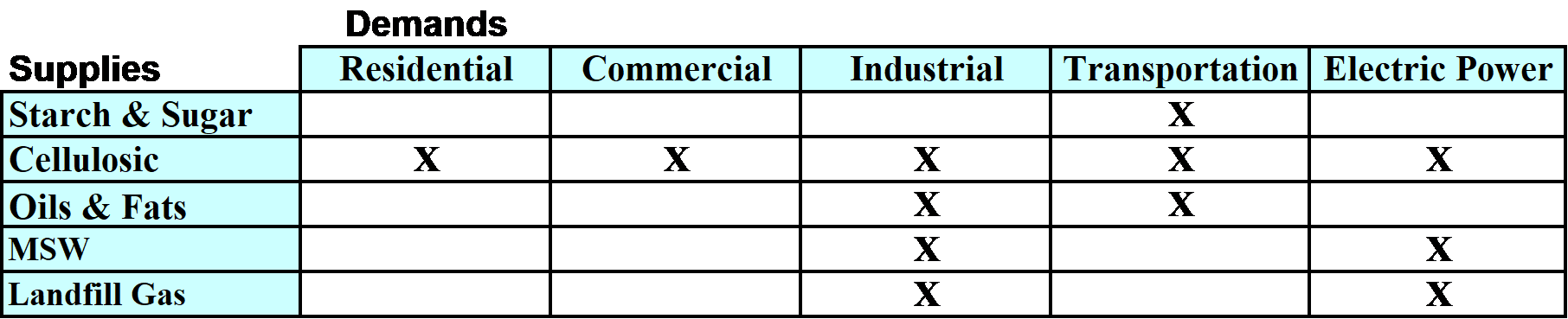

Other
FASOM-GHG is also used to provide estimates of agriculture and forestry GHG abatement supply curves. As such, we are able to model the biomass supply cost implications of GHG incentives for agriculture and forestry. A GHG abatement program that includes agriculture and forestry could provide incentives for changing land management practices, e.g., growing trees, modifying fertilizer use, reducing conventional tillage practices, and reducing livestock methane emissions. Placing a value on GHG reductions could also increase the cost of fossil fuel use and increase the value of bioenergy. We have estimated biomass supplies for scenarios with GHG incentives, and find that the cost of delivered biomass rises notably. Therefore, for proper cost accounting we use these biomass supplies for GHG policy scenarios. Note that, in addition to the adjusted biomass supplies, we also generate complementary agriculture and forests GHG abatement supplies that can be used for GHG policy scenarios.
In addition, to imposing the EISA renewable fuels mandate in FASOM-GHG (noted above), we impose the 2008 Farm Bill's Conservation Reserve Program objective of retaining a minimum of 30 million acres.
See Adams et al. (2009). ↩︎
http://agecon2.tamu.edu/people/faculty/mccarl-bruce/FASOM.htmlopen in new window ↩︎