Buildings
The characterization of building energy in REGEN begins with a detailed calibration exercise to establish how much final energy is consumed by fuel for each end-use, simultaneously with a corresponding allocation of service demand by technology within modeled structural classes. These calculations require reconciliation and adjustments of several distinct but related data sources. In general the model relies on publicly available data from the various products developed by the Energy Information Administration (EIA), including:
State Energy Data System (SEDS), fuel consumption and prices by state, sector, and detailed fuel type, generally not broken out by end-use;
Residential Energy Consumption Survey (RECS), break-down of households by region (2009 edition used more respondents to enable greater geographic resolution, including some individual states; other editions use nine census division resolution), building type, size, and vintage, and fuel/technology equipment type and vintage for space heating and cooling, water heating, and other end-uses;
Commercial Buildings Energy Consumption Survey (CBECS), break-down of commercial buildings by census division, building type, size, and vintage, and fuel/technology equipment type for space heating and cooling, water heating, and other end-uses;
Annual Energy Outlook (AEO), an annual scenario analysis using the National Energy Modeling System (NEMS) that provides additional information on the base year allocation across fuels and end-uses, as well as projections of service demand, at the nine census division level.
To enable state-based model regions in REGEN, the calibration exercise combines these data sources with others (e.g. census population and household estimates and stated-based economic activity from IMPLAN) to down-scale base year results to the state level. Additionally, building stock is allocated to climate zones within each state derived from the historical heating and cooling degree day calculations using gridded weather data from the MERRA2 reanalysis data set maintained by NASA (Figure 3‑2). Note that these climate zones are more finally resolved than the broad categories identified in the RECS and CBECS surveys.
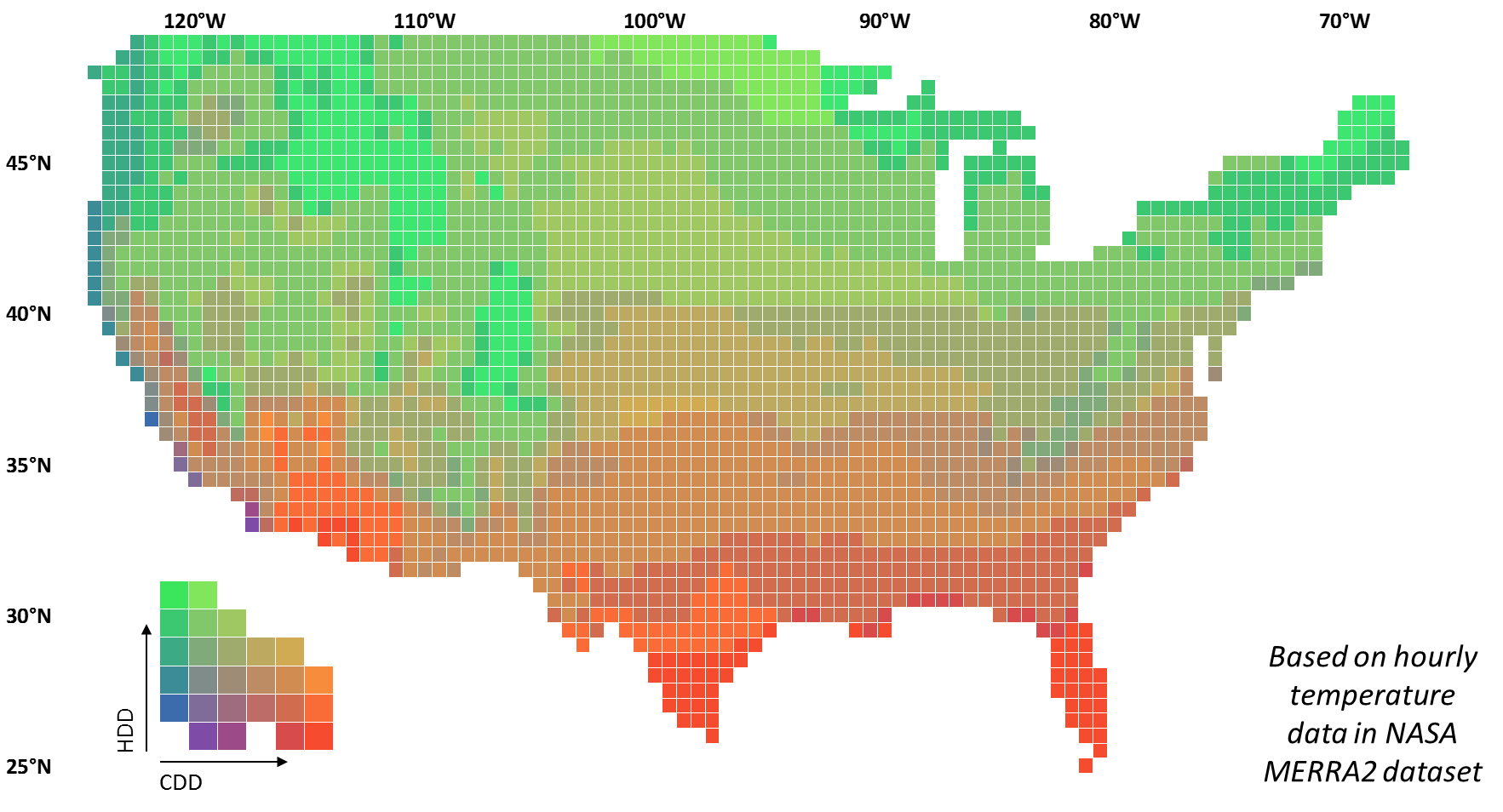
Notes: Heating and Cooling Degree Day zones based on long-run average (1980-2015) temperature profiles in gridded MERRA2 dataset. Heating degrees are calculated relative to 60 deg F, cooling degrees relative to 65 degrees F.
With regard to space cooling and heating and water heating, REGEN uses the EIA's survey data to describe the base year allocation of technologies (e.g. gas furnace vs. heat pump) across the building stock, but it generates its own estimates of base year energy consumption for these end-uses based on its structural characterization of the underlying technologies and hourly temperature profiles from the MERRA2 dataset. This process ensures an internally consistent treatment of the trade-offs between technologies for representative end-use applications.
With the base year established, the model first projects the evolution of future building and equipment vintages based on projected growth rates in households (based on population projections from the US census division and the AEO) and assumed survival rates for buildings and equipment. Buildings are assumed to depreciate at an average annual rate of roughly 1%, with the rate accelerating with building age. For space heating and cooling equipment, roughly 70% is assumed to survive 15 years, while only 3% survives 30 years. Water heating and other equipment is assumed to have a shorter lifetime, such that only 15% survives 15 years. Figure 3‑3 shows the aggregate results of regional projections for US residential building stock in terms of building and space heating/cooling equipment vintages.
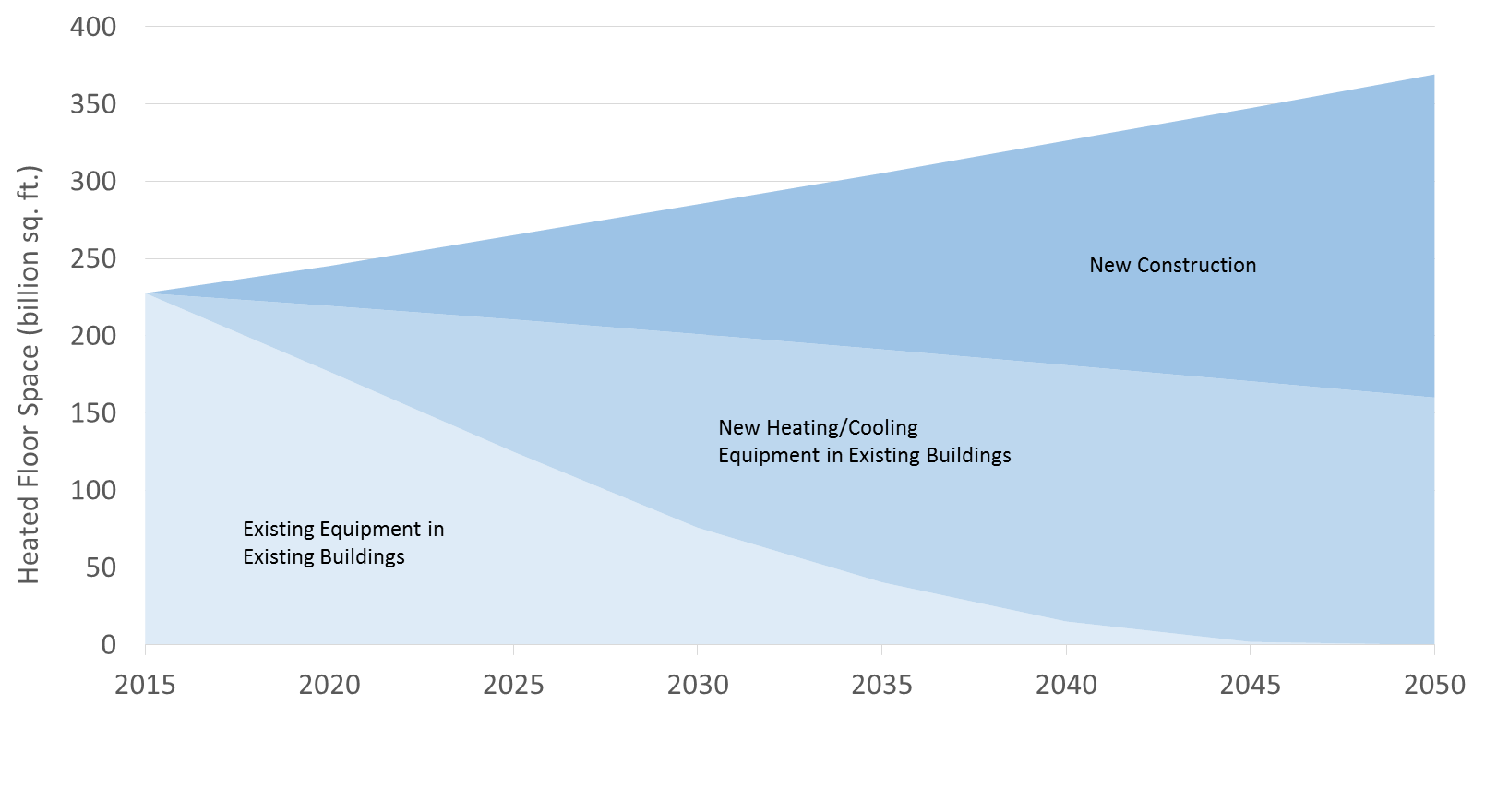
Notes: US Total Residential heated floor space by building vintage and equipment vintage (aggregated into existing vs. new as of model's 2015 base year). Projections vary by region based on age profile and growth rate of total households.
Next, within each vintage, the buildings model evaluates the total cost of each option for (a) space heating and cooling, (b) water heating, (c) cooking, and (d) clothes-dryers. The detailed building model is used to characterize dual-fuel end-uses with explicit technology detail about the cost and performance of the alternative options. Importantly, the break-down into structural categories outlined above informs the cost of each option, in particular for space heating and cooling.
Space Heating and Cooling
Space heating and cooling equipment / technology decisions are modeled jointly to capture in particular the use of heat pumps for both services. For this service, representative residential and commercial buildings are categorized by building vintage, building size, cooling mode, climate zone, and region. Existing building vintages are divided into ten-year categories (e.g. pre-1939, 1940-1949, 1950-1959, etc), while new vintages are assigned for each model projection period (starting in 2020 and progressing in five-year increments). Building size is based on average square footage per residential and commercial building. The model distinguishes two classes in each sector corresponding to small and large, with the national median building size serving as the dividing line. Based on microdata from RECS and CBECS, the median housing unit is 1,670 square feet, and the median commercial building is 50,000 square feet. The model also assumes a heated fraction of total floorspace for each representative building based on the survey data, which is used to scale equipment sizing and energy use. Cooling mode (central, window, or none) is considered a structural class, upon which the composition and costs of heating and cooling equipment in each candidate heating/cooling configuration depends. Heating technology is a "choice class" or decision variable. Alternative options for main-source heating technology include:
- Natural gas furnace
- Oil furnace
- LPG furnace
- Wood-burning furnace or fireplace
- Electric resistance (either furnace, baseboard, or other built-in unit)
- Air-source heat pump with electric resistance back-up
- Air-source heat pump with natural gas back-up
- Air-source heat pump with LPG back-up
- Air-source heat pump with wood-burning back-up
- Ground-source heat pump
- Other (e.g. district heat)
Apart from explicitly modeling the back-up heat source for air-source heat pumps, which is a crucial component of the associated economic trade-offs involved, the model does not track secondary heating equipment, as survey data suggests it contributes little to overall energy consumption.
To assess the total costs of each option, the model calculates (a) the capacity required (i.e. size of equipment) and (b) annual energy consumption for each configuration in each representative structural class. The key parameters for these calculations are the heating/cooling output requirement of the building, the temperature profile of the region / climate zone location, and the performance characteristics of each technology (e.g. energy efficiency).
The energy requirement for heating / cooling in a given hour is assumed to be proportional to both heated / cooled square footage and to heating / cooling degrees for that hour, that is, the difference between the outside temperature and the target temperature. While a more sophisticated building model representation would involve more variables with more complex inter-relationships (such as thermal inertia), this simpler formulation is a reasonable first-order approximation and moreover is necessitated by computational tractability. Whereas detailed building models are typically used to assess the energy profile of a particular building in a particular location, the REGEN end-use model's aim is to simulate aggregate building energy use across many representative building types spanning the continental US and extending several decades into the future under various scenarios.
One important element of more detailed building simulations is the quantification of internal gains, that is, heat from the building itself and its occupants and non-space heating energy-using equipment. To account for internal gains implicitly, REGEN measures cooling degrees relative to 65 degrees and heating degrees relative to 60 degrees (both Fahrenheit). For example, if internal gains contribute 7 degrees of space heating, these target temperatures correspond to a target indoor temperature range of 67-72 degrees.
The choice of the scaling coefficient relating heating and cooling degrees to energy requirement per square foot of floorspace is essentially a calibration parameter, but it varies by building type (residential vs. commercial), building size, building vintage (and time step), and climate zone. First, cooling energy requirement per sq.ft.-degree-hour is observed to be greater than heating energy requirement, particularly in commercial buildings. Residential buildings are observed in the RECS and CBECS data to consume less energy per sq.ft.-degree-hour than commercial buildings for both heating and cooling, all else equal. Smaller housing units are assumed to consume more energy per sq.ft.-degree-hour due to the different relationship between perimeter and area. The coefficients used for each of these structural classes are summarized in Table 3‑1.
Heating | Cooling | |
---|---|---|
Residential (Large) | 0.20 | 0.29 |
Residential (Small) | 0.31 | 0.45 |
Commercial | 0.40 | 0.80 |
Notes: Coefficients for building space heating and cooling requirement are expressed in btu per square-foot-degree F per hour for the nominal case of new construction building in 2015 in mildest climate zone.
Additionally, older buildings are assumed to have lower shell efficiency and thus consume more energy per sq.ft.-degree-hour, although all building vintages are assumed to improve over time through shell retrofits such as insulation and window replacement. New construction building shell efficiency improves at a rate of 0.5% per year, while existing building shell efficiency improves at a rate of 0.25% per year. For example, a building built in the 1970's is assumed to use 20% more energy than a new construction building in 2015, and a new construction building in 2045 is assumed to use 20% less energy, all else equal. Finally, buildings in colder climates are observed to have better shell efficiency, such that the energy consumption per sq.ft.-degree-hour in the coldest climates is 25% lower than in the mildest climates.
Technology performance is characterized by a coefficient relating heating / cooling energy output to energy input, known as the coefficient of performance (COP). For non-electric heating technologies, the COP is a single number, which is assumed to improve by equipment vintage through 2030, after which point it remains constant (See Table 3‑2). Electric resistance heating is assumed to be 100% efficient (that is, all electric energy consumed by the equipment is converted to heat) in all vintages.
1989 | 1999 | 2009 | 2015 | 2020 | 2025 | 2030 | |
---|---|---|---|---|---|---|---|
Natural Gas | 0.75 | 0.78 | 0.80 | 0.83 | 0.85 | 0.87 | 0.89 |
Oil / LPG | 0.71 | 0.74 | 0.76 | 0.79 | 0.81 | 0.83 | 0.85 |
Wood | 0.68 | 0.70 | 0.72 | 0.75 | 0.77 | 0.79 | 0.81 |
Notes: Coefficient of performance (energy output per energy input) for non-electric heating technologies by equipment vintage. These numbers reflect stock average equipment efficiency.
The performance of air-source heat pumps depends on the outside temperature. As the gap between the outside and insider temperature increases, both the efficiency (i.e. COP) and the capacity (i.e. potential output) of the heat pump decline. Additionally, heat pump efficiency is reduced by cycling or operation at partial load factors. While the loss of performance as a function of temperature decline is based on fundamental thermodynamic properties of heat pump technology, improvements in technology can shift these curves over time. REGEN assumes improvements over time in both the COP-temperature curve and the cycling losses, while the relationship between capacity and temperature is assumed to remain constant over time (see Figure 3‑4, Figure 3‑5, and Figure 3‑6). One key technological improvement assumed to underlie these shifts is the use of variable speed motors, which reduce cycling losses and improve efficiency in milder temperatures, enabling larger capacity heat pumps to provide more energy in colder temperatures.
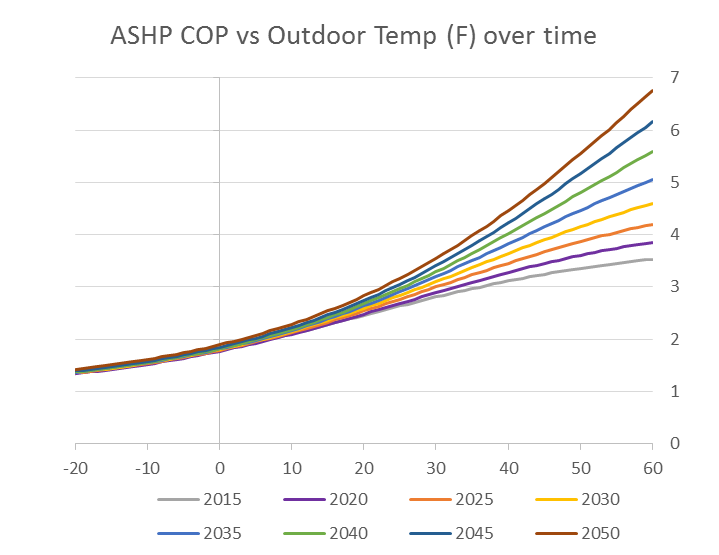
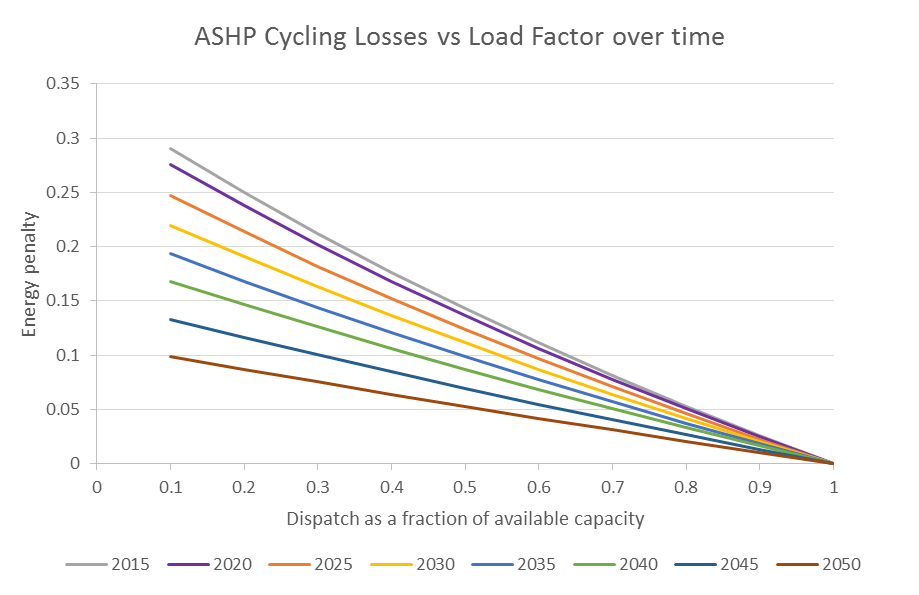
Notes: Heat pump capacity expressed relative to nominal rated capacity.
Determining Equipment Capacity
For technologies not involving an air-source heat pump, the determination of required capacity in each representative building type is straightforward. For each technology we assume a design cycling factor in peak operating conditions. For non-electric heat sources, this parameter is 0.5, e.g. we assume that a gas furnace is sized so that it runs 50% of the time during the coldest hour. For electric resistance heating, this parameter is assumed to be 1, that is, sized so that it is running 100% of the time during the coldest hour. The maximum heating output requirement is calculated for each representative building type and each climate zone as the product of the long-run maximum heating degree hour, the square footage of the unit, and the coefficient relating btus to sq.f.t.-degree-hour. Then the equipment size is determined by dividing the required output by the cycling factor.
For example, in a region with a minimum temperature of -20 degrees F, a 2,000 sq.ft. residential building with shell heating efficiency of 0.2 btu per sq.ft.-degree-hour would have a maximum output requirement of 80 heating degrees * 2000 sq.ft. * 0.2 btu per sq.ft.-degree-hour = 32,000 btu per hour. Dividing by 0.5, this building would require a gas furnace of output capacity 64,000 btu per hour, or an electric-resistance furnace rated at 32,000 btu per hour = 9.4 kW.
For air-source heat pumps, the sizing calculation is more complicated. An additional decision must be made about the relative size of the heat pump vs. its back-up heat source. Ideally this decision would be made based on an optimization of the trade-off between size of equipment and performance efficiency given the building and climate characteristics, cost structure of the equipment capacity, and fuel prices. REGEN can be configured to determine optimal heat pump capacity based on such a formulation, however it is highly computationally intensive given the high dimensionality of the structural classes into which the building stock is disaggregated. For most scenarios, a rule of thumb is used instead whereby the capacity of the heat pump is determined by the maximum cooling load for the representative building. Because the same piece of equipment is used for both heating and cooling in heat pump configurations, the capacity must be at least as large as the maximum cooling load. If this proves to be a binding constraint in the full sizing optimization, then this rule of thumb corresponds to the optimal size. Generally, the cooling load is the binding constraint in warmer climates where heat pumps are already beginning to deploy. In cooler climates with lower maximum temperatures, it may in fact be optimal to over-size the heat pump relative to required cooling load, depending on other conditions and parameters. This case can be explored further with the more detailed optimization formulation. As a proxy for a heat pump system optimized for cold climates, the model includes a configuration in which the heat pump's capacity is twice as large as required for cooling. In this configuration, the capital costs are higher, but the need for electric resistance backup is significantly reduced or in some cases eliminated.
Cooling capacity is determined in an analogous fashion to heating capacity as described above, with the additional factor that cooling capacity declines as temperature rises, for the same reason that air conditioners and heat pumps become less efficient as the temperature differential increases. The cooling COP for heat pumps and air conditioners is assumed to improve over time, with an average new vintage SEER rating rising from around 12 in 2015 to 19 in 2050. These relationships are summarized in Figure 3‑7 and Figure 3‑8.
For example, in a region with a maximum temperature of 105 degrees (at which the capacity is assumed to be 0.95 relative to nominal), a 2,000 sq.ft. residential building with shell cooling efficiency of 0.29 btu per sq.ft.-degree-hour would have a maximum output requirement of 40 cooling degrees * 2000 sq.ft. * 0.29 btu per sq.ft.-degree-hour / 0.95 = 24,000 btu per hour (approximately). This corresponds to a two-ton nominal rating for a heat pump.
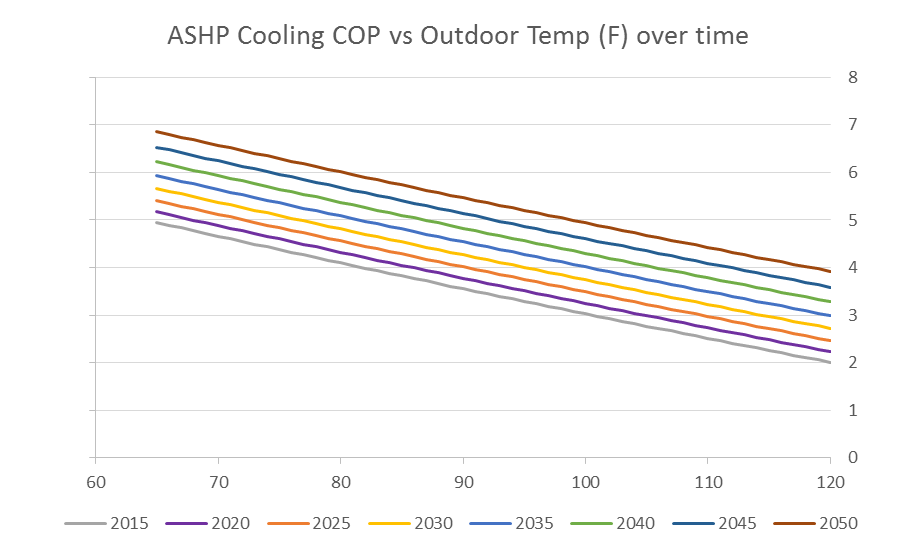
Notes: Air-source heat pump / Central air-conditioner cooling COP vs. temperature by technology vintage.
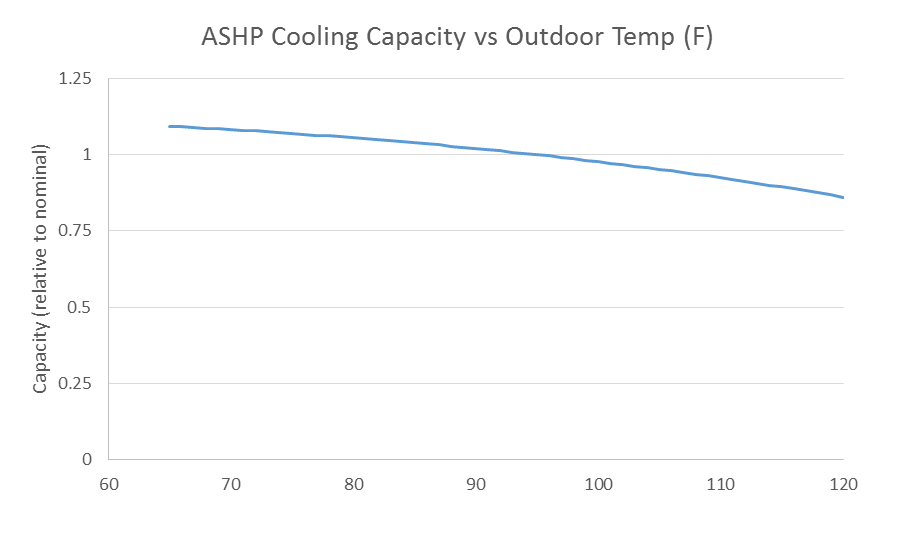
Notes: Air-source heat pump / Central air-conditioner cooling capacity (relative to nominal rated capacity) vs. temperature by technology vintage.
Once the heat pump capacity is calculated based on cooling load, or in the cold-climate configuration as twice cooling load, the size of the back-up heating capacity is determined based on the residual heating requirement after the contribution of the heat pump at the long-run minimum temperature. That is, the heat pump is assumed to continue to operate as the temperature falls, albeit with diminished capacity. One exception is the configuration pairing a heat pump with natural gas back-up, in which the heat pump is assumed to shut off when the gas back-up is in use.
For example, in a region with a minimum temperature of 0 degrees (at which the capacity is assumed to be 0.5 relative to nominal), and heat pump sized to 24,000 btu per hour as above, a 2,000 sq.ft. home with shell heating efficiency of 0.2 btu per sq.ft.-degree-hour would have a maximum total output requirement of 60 heating degrees * 2000 sq.ft. * 0.2 btu per sq.ft.-degree-hour = 24,000 btu per hour. The heat pump operating at 0.5 nominal capacity would contribute 12,000 btu per hour, so the back-up heating source would need to provide an additional 12,000 btu per hour. For an electric resistance back-up, this corresponds to 3.5 kW of capacity. On the other hand a natural gas back-up would need to supply all 24,000 btu per hour, again divided by the 0.5 cycling factor for gas, implying a nominal capacity of 48,000 btu per hour.
Determining Annual Energy Use
The model next calculates space heating and cooling energy use in each candidate technology configuration across a range of temperature segments. These segments correspond to 5-degree bins, and from the MERRA2 historical hourly temperature data is derived a distribution profile across theses bins in each region and climate zone. Integrating the simulated energy consumption in each temperature segments with the distribution profile across the segments provides a good approximation of annual energy consumption in each configuration. This approach is a less computationally intensive alternative to calculating energy use in every hour of a representative year across all structural building classes and candidate technology configurations.
For non-heat-pump configurations, energy use for a given temperature segment is straightforward, because the coefficient of performance does not depend on the outdoor temperature. For these technologies, energy use is simply output divided by COP, where output is determined as above using the median temperature in the segment.
For heat pump energy consumption, the model first determines the load factor for the heat pump in the given segment, based on available capacity versus required output. As soon as required output exceeds available capacity, the remaining output is met using the back-up source. Thus the "crossover point" at which the back-up begins to dispatch is determined differently for each structural building class based on its parameterization, sizing of cooling load, shell efficiency, etc. Again, with the natural gas back-up, in all temperature segments below which the heat pump is not able to meet required output, it is not dispatched at all, with the gas back-up provided all required output. Energy consumption is equal to output divided by COP (dependent on temperature), and scaled by the cycling penalty based on the load factor (which reduces to 1 when the heat pump is operating at full load).
An additional wrinkle is that the model does not dispatch the heat pump ahead of the gas back-up in a temperature segment if the marginal cost of the electricity consumption exceeds that of the gas consumption required to run the gas back-up. That is, the fuel prices and other parameters may be such that in some circumstances, the "crossover point" is higher than the point at which heat pump capacity becomes insufficient. Moreover, if the marginal energy cost of gas is lower than that of the heat pump for all heating segments, the heat pump + gas backup configuration reverts to a conventional gas furnace + central A/C configuration.
Determining Total Cost of Each Configuration
With capacity and energy use quantified for each candidate configuration, the model calculates the total cost of the equipment plus operating costs for energy and maintenance. Equipment and installation costs are based on the individual components that make up each configuration. Cost assumptions are made for each component, with labor and capital costs expressed as linear functions of size (i.e. capacity) with a non-zero intercept. That is, there is a certain cost for each component that does not scale with the size of the component. Additionally there is a slope term that reflects the marginal cost of increasing the size. This relatively simple formulation reflects the observation that for heating and cooling equipment, marginal cost is typically less than average cost per nominal btu of output. Cost parameters have been estimated using the RSMeans database. Additionally, a retail markup is applied to capture regional variation in vendor costs. The national average markups for capital (i.e. materials) and labor costs are 10% and 65%, respectively. Table 3‑3 lists the individual components and their assumed cost structure. Table 3‑4 shows the mapping between a selection of heating / cooling technology configurations and the components involved. Note that all costs are assumed to remain constant over time, with the exception of the "reverse valve" component. This component essentially reflects the cost difference between a heat pump designed to provide cooling and heating and an otherwise equivalent central air-conditioner unit. This cost difference is expected to decline over time.
Capital Fixed Cost | Capital Marginal Cost (per 000 btu) | Labor Fixed Cost | Labor Marginal Cost (per 000 btu) | |
---|---|---|---|---|
Furnace (with blower) | 540 | 2 | 120 | 0.7 |
Outdoor heat exchanger | 520 | 30 | 0 | 15 |
plus reverse valve | 500 | 0 | 0 | 2 |
Air handler (coil + blower) | 408 | 12 | 100 | 2 |
Separate coil | 40 | 10 | 100 | 2 |
Separate blower | 368 | 2 | 100 | 2 |
Heat Strips | 50 | 2 | 0 | 1 |
Ground-source loop | 6000 | 100 | 0 | 50 |
Furnace (with blower) | Outdoor heat exchanger | plus reverse valve | Air handler (coil + blower) | Separate coil | Separate blower | Heat Strips | Ground-source Loop | |
---|---|---|---|---|---|---|---|---|
Non-electric heat / no cooling | x | |||||||
Non-electric heat / central A/C | x | x | x | |||||
ASHP / resistance back-up | x | x | x | x | ||||
ASHP / non-electric back-up | x | x | x | x | ||||
Electric resistance heat / no cooling | x | x | ||||||
Electric resistance heat / central A/C | x | x | x | |||||
No heat / central A/C | x | x | ||||||
Ground-source heat pump | x | x | x | x |
Total equipment cost is calculated by integrating the mapped components with each component's cost function applied to the calculated size (i.e. capacity) for each candidate configuration in each structural building class. This total capital cost is annualized by calculating a capital recovery factor assuming a discount rate and investment lifetime. Typically these values are set to 7% and 20 years, respectively. However, other assumptions can be used for alternative scenarios. Additionally, the model assumes a fixed operating and maintenance cost of 2% of the total capital cost per year. Energy costs are straightforward based on calculated annual energy consumption and assumed retail fuel prices for the year in which the vintage is being installed. Alternative assumptions could allow for foresight of projected prices over the lifetime of the vintage.
Logit Model
In each structural building class, a total annualized cost metric has been derived for each candidate configuration based on structural attributes and technology characterization. Next, a nested logit model is used to translate these relative costs into potential market share for the candidate heating configurations. The logit model is characterized by (a) the nest structure, and (b) the logit parameter assigned to each nest. Figure 3‑9 shows the nesting structure and corresponding logit parameters for space heating configurations.
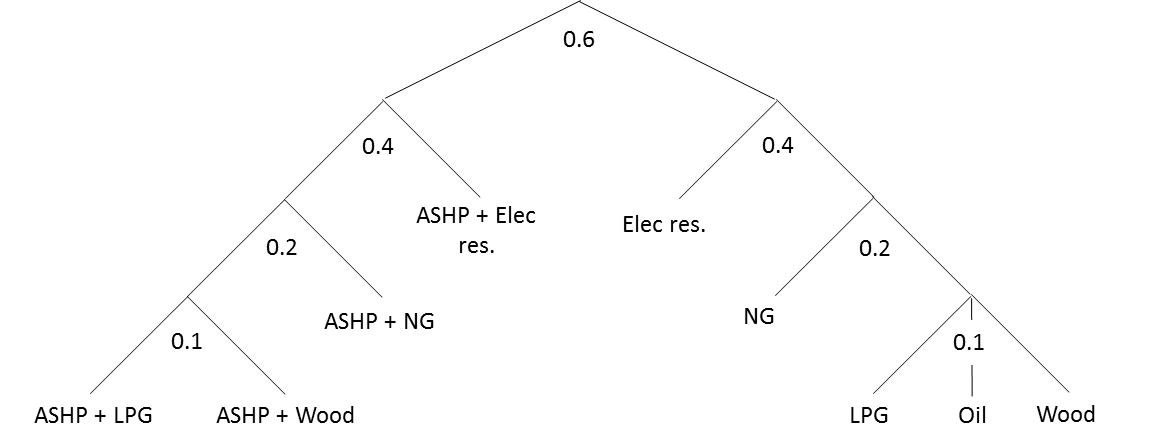
Note that annualized costs are expressed in units of thousands of dollars per representative housing unit. The logit parameters can be interpreted (in the same units) as the magnitude of a randomly distributed unobserved additional cost component. Based on the statistical properties assumed for these implicit unobserved costs, the logit model calculates the probability that each candidate is the lowest cost among the options within its nest. These probabilities are interpreted as potential or equilibrium market shares for each candidate option. That is, supposing the economic conditions giving rise to the annualized cost metrics assigned to each candidate were to persist indefinitely, the allocation of market choice across the candidates (for new vintages) would equilibrate according to the calculated shares. However, these shares may be significantly different than the realized shares in the previous vintage. Market choice, even for new vintage decisions, may not evolve as quickly as the underlying technology. To account for this, a lag parameter is introduced, so that realized market shares in a given time step are a linear combination of the realized shares in the previous step and the potential equilibrium shares calculated in the current step. The lag parameter determines the relative weighting on the previous step vs. the current potential. It is characterized as an annual rate at which the market moves from its current state toward the new equilibrium. If this rate is 0, the market never evolves from its current allocation. If this rate is 1, the market immediately shifts to the new equilibrium. In the current version of REGEN, a rate of 0.1 is assumed for space heating and cooling equipment in existing buildings (i.e. retrofit or replacement), and a rate of 0.2 is assumed for space heating and cooling equipment in new construction. That is, equipment configuration in new construction is expected to adapt more quickly to changing techno-economic conditions because new designs can be incorporated more easily into new structures. For five-year time steps, these rates imply a weight of approximately 0.6 and approximately 0.3 on the previous vintage shares in existing buildings and new construction, respectively. A conceptual diagram of the lagged nested logit model approach is depicted in Figure 3‑10.
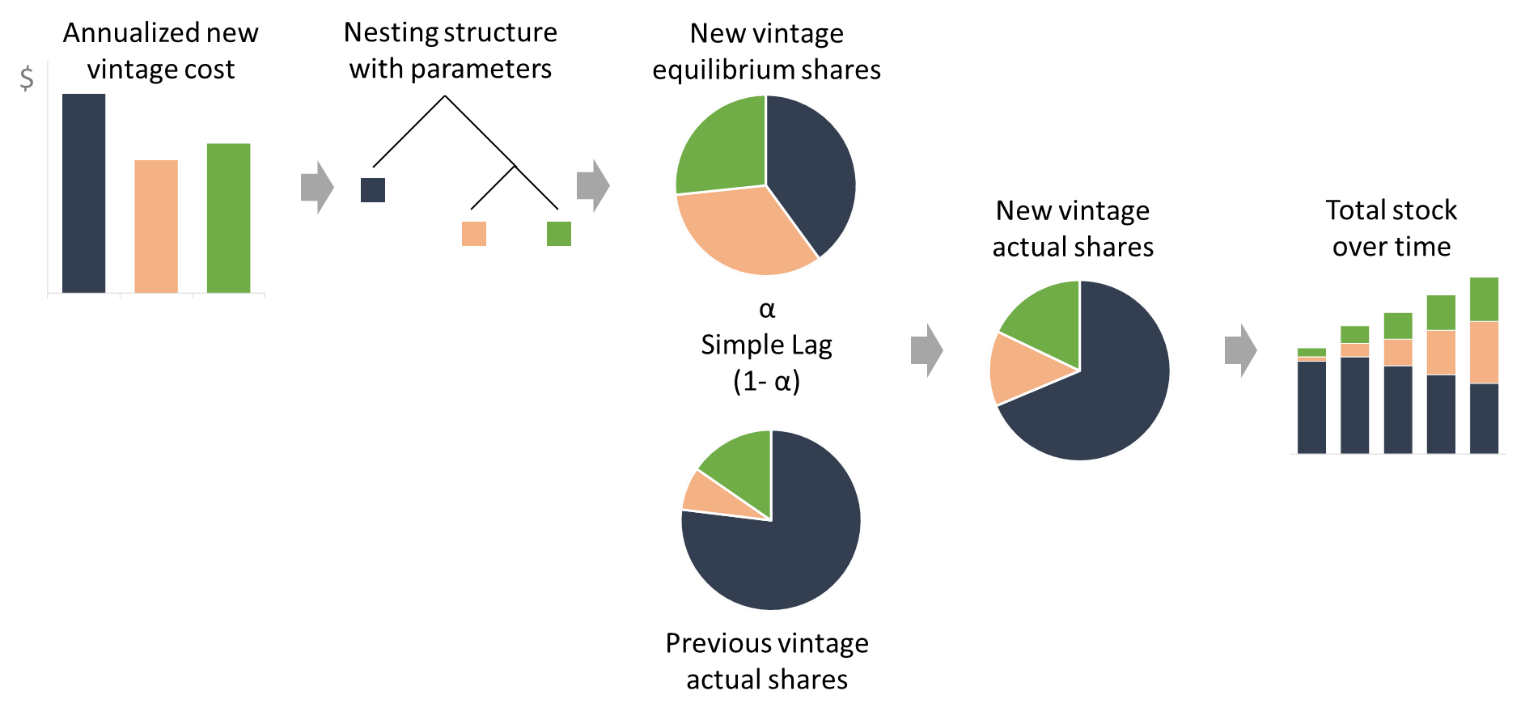
Water Heating, Cooking, and Clothes Dryers
A similar approach is used for other dual-fuel building uses, although with less structural disaggregation. Only one representative building is considered per region, although equipment vintages are tracked explicitly. Energy consumption is based on the national average per household consumption for these uses according to RECS. For water heating, average groundwater temperature varies by region, which affects the energy required per service unit. For each model region, annual mmbtu per household is scaled from the national average according to the difference between the target hot water temperature of 150 degrees F and the average groundwater temperature. For example, a region with an average groundwater temperature of 50 degrees F would use 25% more energy per household for water-heating than a region with 70 degree groundwater (assuming the same technology). Figure 3‑11 shows variation in groundwater temperature in the US.
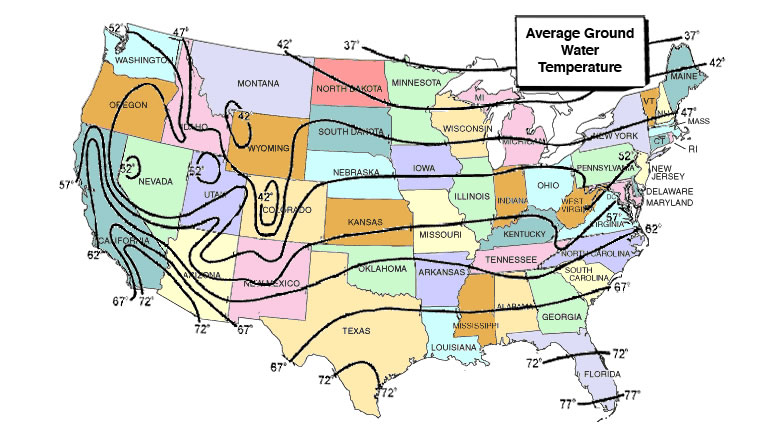
Candidate technologies correspond to alternative fuel choices, and each is characterized by a capital cost for equipment and an annual energy use per household by vintage. The latter is derived from RECS for existing vintages. Table 3‑5 summarizes these assumptions. The capital recovery factor to annualize equipment cost is based on 7% discount rate and investment lifetime to 10 years. A similar lagged nested logit model is applied to each of these end-uses. The nesting structure and corresponding logit parameters are depicted in Figure 3‑12. Costs are again expressed in terms of thousands of dollars per housing unit. The lag parameter is derived from an assumed annual rate of convergence toward potential equilibrium shares of 0.1.
Water Heating | Equipment cost | Annual energy use (mmbtu) |
---|---|---|
Natural Gas | $600 | 20.2 |
Oil | $600 | 20.2 |
Electric resistance | $500 | 14.1 |
Electric heat pump | $1,400 | 5.7 |
Water heating notes: Electric resistance is assumed to be 30% as efficient as non-electric on a btu basis. Electric heat pump is assumed to be 2.4x as efficient as electric resistance. All improve at a rate of 0.5% for future vintages. Electric heat pump water heater costs also decline at 0.5% per year.
Cooking | Equipment cost | Annual energy use (mmbtu) |
---|---|---|
Natural Gas | $1,000 | 5.29 |
Oil / LPG | $1,000 | 5.29 |
Electric | $850 | 2.46 |
Cooking notes: Electric is assumed to be twice as efficient as non-electric on a btu basis. Both improve at an annual rate of 0.5% for future vintages. Note that induction electric is not explicitly considered.
Clothes-dryers | Equipment cost | Annual energy use (mmbtu) |
---|---|---|
Natural Gas | $1,200 | 2.92 |
Electric | $900 | 2.66 |
Clothes-dryers notes: Electric is assumed to be 10% more efficient on a btu basis. Both improve at an annual rate of 0.5% for future vintages.
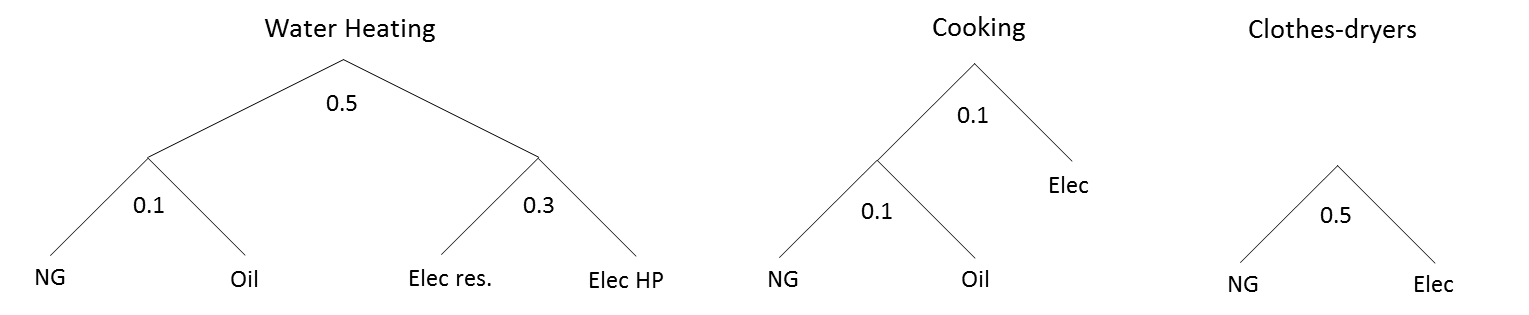
Other All-Electric Building Uses
Less technological detail is employed to represent all-electric building uses (apart from cooling) such as lighting, electronics, appliances (apart from dual-fuel cooking and clothes-dryers), ventilation, and other miscellaneous uses. Because these uses do not involve a trade-off between fuels, the main modeling questions are around service demand growth and changes in energy efficiency (energy consumed per service unit). Projections for service demand are driven by floorspace projections, with some services growing slightly faster (e.g. lighting and electronics) and some growing more slowly (e.g. appliances). Total floorspace is projected based on AEO trends for total households, increasing floorspace per household, and commercial floorspace. Projections for energy efficiency for each end-use are based on assumed technology trends (such as more widespread LED adoption) and continued improvements, particularly driven by digitization and enhanced controls. Table 3‑6 shows the assumed rates of improvement for key all-electric building end-uses. The model also includes an option to accelerate the rate of improvement in end-use efficiency as energy prices rise by investing more capital.
Annual rate | |
---|---|
Lighting (residential) | 4.0% |
Lighting (commercial) | 3.0% |
Electronics (including TVs, computers, IT equipment, etc.) | 2.0% |
Appliances (including refrigerators, dishwashers, etc.) | 1.5% |
Others (including ventilation, miscellaneous uses) | 0.5% |