Renewable Resources
Wind and Solar
US-REGEN represents wind and solar energy resources based on a detailed characterization of their spatial and temporal variation and potential scale. Variability is modeled using gridded hourly data from the NASA MERRA2 dataset. This dataset provides key meteorological variables such as wind speed, solar irradiation, and temperature, from 1980 to the present. While long-run averages are used to characterize grid cells in terms of resource quality, hourly profiles used in the model solution are based on a single representative year, typically 2015. This is the same base year as the corresponding load data, or the temperature data used to derive load in the end use model, to ensure synchronicity between wind, solar, and load and to avoid damping variance through multi-year averaging. The model does have the capability of varying the representative year to explore the implications of inter-annual variation.
Potential capacity is estimated based on a state-level dataset developed for EPRI by AWS Truepower (2012). The AWS dataset was developed using a screening process to determine the amount of land area available for wind and solar installations in each state. This screening process disallowed siting in areas such as National Parks, excessively sloping land, and developed lands. For wind, total potential capacity was calculated as the sum of potential facility siting locations. For solar, available land was ranked by resource quality, and potential capacity was approximated based on the top 1% of this available land.
Wind Resources
Wind output profiles were derived from 2015 gridded hourly wind speed data from the MERRA-2 reanalysis dataset. We begin with the MERRA-2 variables referring to the east/west and north/south components of the wind speed vector at 50 meters, which are combined into a single vector extrapolated to various alternative hub heights using the MERRA-2 variable referring to the surface roughness coefficient, following the Monin-Obukhov specification.[1] Figure 2‑6 shows the long-run average wind speed at 100m for each grid cell, which were used to form classes based on resource quality. The number of grid cells in each class, combined with the state-level potential assessment from AWS Truepower, was used to derive total potential GW of wind capacity by resource class and state, shown in Figure 2‑7. Expand the "Wind Resource Class Definitions and Data Table" panel below Figure 2-7 to see details about the data.
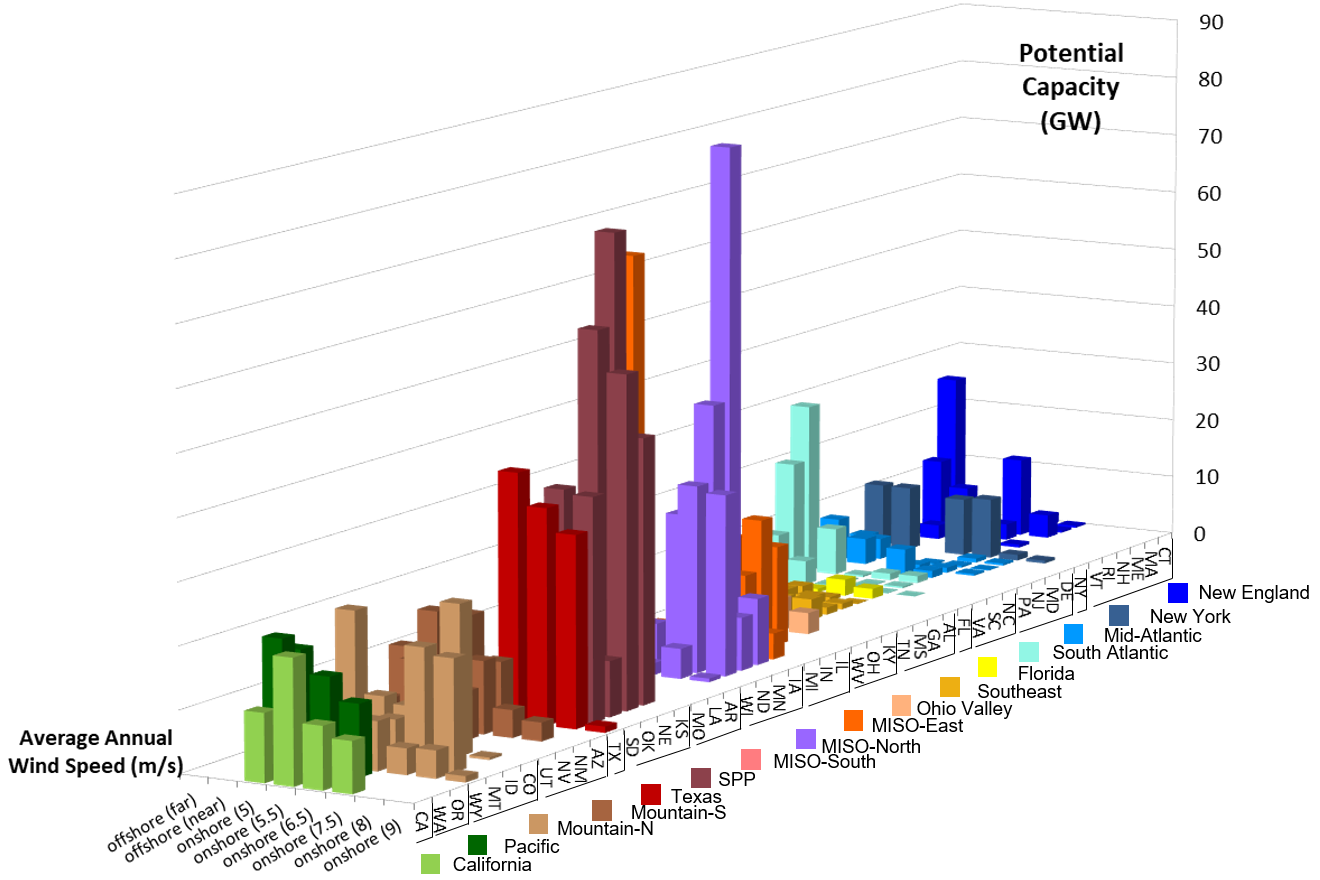
Wind Resource Class Definitions and Data Table
- Onshore (9): Onshore wind with long-run average speed at 100m btw 8.25 and 9.5 m/s
- Onshore (8): Onshore wind with long-run average speed at 100m btw 7.75 and 8.25 m/s
- Onshore (7.5): Onshore wind with long-run average speed at 100m btw 7.25 and 7.75 m/s
- Onshore (6.5): Onshore wind with long-run average speed at 100m btw 6.25 and 7.25 m/s
- Onshore (5.5): Onshore wind with long-run average speed at 100m btw 5.25 and 6.25 m/s
- Onshore (5): Onshore wind with long-run average speed at 100m less than 5.25 m/s
- Offshore (near): Offshore wind within 50 km from shore
- Offshore (far): Offshore wind btw 50 and 100 km from shore
Data Table (GW potential in each resource class):
Region | Onshore (9) | Onshore (8) | Onshore (7.5) | Onshore (6.5) | Onshore (5.5) | Onshore (5) | Offshore (near) | Offshore (far) |
---|---|---|---|---|---|---|---|---|
NewEngland | 5.10 | 23.25 | 9.52 | 38.70 | ||||
NewYork | 0.48 | 0.96 | 10.05 | 9.57 | 10.60 | 10.60 | ||
MidAtlantic | 0.72 | 3.02 | 5.42 | 7.76 | 12.24 | |||
SouthAtlantic | 0.04 | 1.52 | 1.78 | 0.82 | 11.71 | 55.60 | ||
Southeast | 5.69 | 6.98 | 8.37 | |||||
Florida | 1.67 | 2.71 | 0.63 | |||||
OhioValley | 3.52 | 6.68 | 5.87 | 2.05 | 7.94 | |||
MISO-East | 4.27 | 51.21 | 40.44 | 1.67 | 65.74 | 5.85 | ||
MISO-North | 49.87 | 163.00 | 26.54 | 6.15 | 14.21 | |||
MISO-South | 7.57 | 26.42 | 29.23 | 10.75 | ||||
SPP | 142.48 | 187.58 | 11.66 | 1.73 | ||||
Texas | 1.00 | 31.04 | 34.80 | 39.96 | 3.44 | 3.87 | ||
Mountain-N | 1.00 | 22.74 | 47.33 | 28.84 | 22.99 | 35.96 | ||
Mountain-S | 3.02 | 4.44 | 36.00 | 53.62 | 22.48 | |||
Pacific | 15.99 | 20.12 | 40.56 | |||||
California | 8.28 | 10.08 | 20.10 | 10.97 |
Solar Resources
Solar output profiles were derived from gridded hourly radiation flux data from the MERRA-2 reanalysis dataset. We begin with the MERRA-2 variable referring to surface incident shortwave radiative flux, which we interpret as the global horizontal irradiance (GHI) in each hour / grid cell. From this flux we derive two related quantities, the diffuse component of GHI and the corresponding direct normal irradiance (essentially the direct component of GHI adjusted by the angle of incidence). The diffuse share is estimated by comparing GHI to a hypothetical horizontal flux at the top of the atmosphere, which is calculated based on the angle of the sun in a given hour and the coordinates of the grid cell. This ratio, always less than (or equal to) 1, is interpreted as a clearness index and is used to estimate the diffuse component. Essentially, the lower the clearness index, the higher the share of surface incident irradiance that is assumed to be diffuse. The estimation function is based on Ridley et al (2010). Once the diffuse component is estimated, the direct horizontal component is calculated as the difference between GHI and the diffuse component, and DNI is subsequently calculated by adjusting for the angle of incidence. DNI is always greater than (or equal to) the direct horizontal component because it reflects irradiance incident to a plane perpendicular to the incoming flux. Figure 2‑9 shows the long-run average GHI for each grid cell, which were used to form classes based on resource quality. The number of grid cells in each class, combined with the state-level potential assessment from AWS Truepower, was used to derive total potential GW of solar capacity by resource class and state, shown in Figure 2‑10. Expand the "Solar Resource Class Definitions and Data Table" panel below Figure 2-10 to see details about the data.
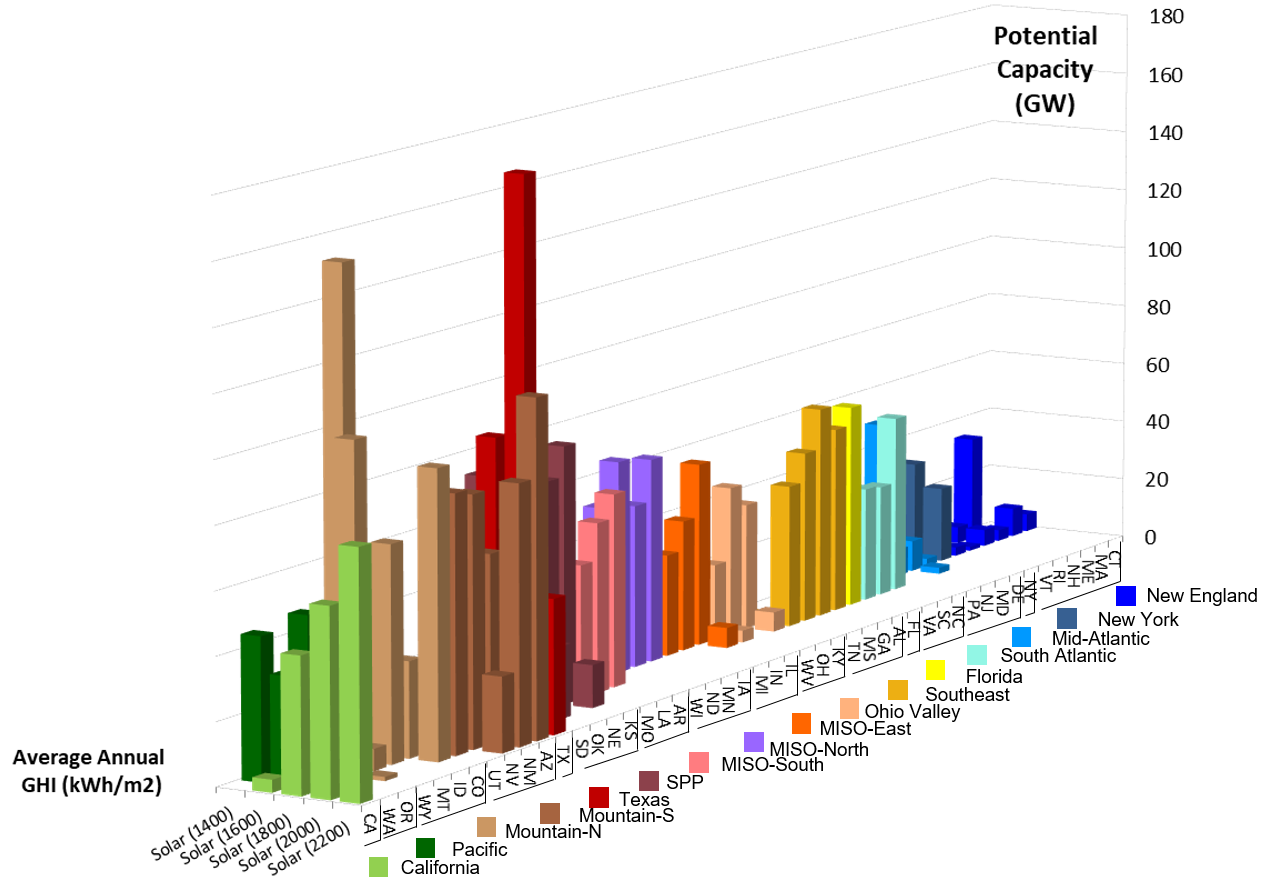
Solar Resource Class Definitions and Data Table
- Solar (2200): Long-run average GHI btw 2100 and 2300 kWh/m2
- Solar (2000): Long-run average GHI btw 1900 and 2100 kWh/m2
- Solar (1800): Long-run average GHI btw 1700 and 1900 kWh/m2
- Solar (1600): Long-run average GHI btw 1500 and 1700 kWh/m2
- Solar (1400): Long-run average GHI less than 1500 kWh/m2
Data Table (GW potential in each resource class):
Region | Solar (2200) | Solar (2000) | Solar (1800) | Solar (1600) | Solar (1400) |
---|---|---|---|---|---|
NewEngland | 28.39 | 48.02 | |||
NewYork | 24.64 | 32.03 | |||
MidAtlantic | 2.01 | 74.00 | |||
SouthAtlantic | 130.62 | 10.09 | |||
Southeast | 230.54 | 2.89 | |||
Florida | 65.99 | ||||
OhioValley | 10.13 | 113.29 | |||
MISO-East | 6.57 | 134.03 | 34.95 | ||
MISO-North | 240.75 | 70.92 | |||
MISO-South | 159.12 | 38.55 | |||
SPP | 36.78 | 226.42 | 94.19 | ||
Texas | 42.85 | 174.26 | 91.41 | ||
Mountain-N | 92.29 | 209.18 | 180.79 | 21.85 | |
Mountain-S | 213.70 | 246.04 | 42.65 | ||
Pacific | 44.22 | 84.49 | 63.24 | ||
California | 78.03 | 59.20 | 43.05 | 4.04 |
Hydro and Geothermal Resources
US-REGEN assumptions for hydro and geothermal resources are documented in the corresponding section under Electricity Generation > Hydro and Geothermal.
The implication of Monin-Obukhov Similarity Theory is that wind speeds at different layers in the atmosphere are related according to:
speed(h) = speed(50) * ln(h / z0) / ln(50 / z0)
, whereh
refers to hub height in meters andz0
refers to the surface roughness coefficient for the grid cell. The smoother the surface, the less the relative change in wind speed at higher layers. ↩︎